Forthcoming and Online First Articles
International Journal of Swarm Intelligence
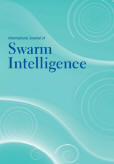
There are currently no forthcoming papers for this journal.
Register for our alerting service, which notifies you by email when new issues are published online.
There are currently no forthcoming papers for this journal.
Register for our alerting service, which notifies you by email when new issues are published online.