Forthcoming and Online First Articles
International Journal of Information and Communication Technology
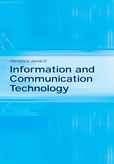
Forthcoming articles have been peer-reviewed and accepted for publication but are pending final changes, are not yet published and may not appear here in their final order of publication until they are assigned to issues. Therefore, the content conforms to our standards but the presentation (e.g. typesetting and proof-reading) is not necessarily up to the Inderscience standard. Additionally, titles, authors, abstracts and keywords may change before publication. Articles will not be published until the final proofs are validated by their authors.
Forthcoming articles must be purchased for the purposes of research, teaching and private study only. These articles can be cited using the expression "in press". For example: Smith, J. (in press). Article Title. Journal Title.
Articles marked with this shopping trolley icon are available for purchase - click on the icon to send an email request to purchase.
Online First articles are published online here, before they appear in a journal issue. Online First articles are fully citeable, complete with a DOI. They can be cited, read, and downloaded. Online First articles are published as Open Access (OA) articles to make the latest research available as early as possible.
Register for our alerting service, which notifies you by email when new issues are published online.
International Journal of Information and Communication Technology (10 papers in press) Regular Issues
Abstract: The signal of intelligent nursing bed is easily polluted by noise during the acquisition process, so it is necessary to study the noise reduction processing algorithm of the signal. This paper uses deep learning to optimise the bowel sound feature extractor, takes the edge computing system with GPU configuration as the implementation object, and proposes a pre-defecation prediction based on Mobilenet-RF. This paper proposes to use the random forest algorithm to classify the bowel sound signal features extracted by Mobilenet to achieve early classification and prediction of patient characteristics. Furthermore, this paper uses bowel sound signal processing and pre-defecation prediction as cases for experimental analysis. The experimental results show that the Mobilenet-RF algorithm proposed in this paper achieves the highest accuracy of 95.68%. Then, this paper verifies the generalisation ability of the model through multi-dataset experiments, proving the superiority of using random forest for classification. Keywords: intelligent nursing bed; patient; signals; feature extraction.
Abstract: As information technology and artificial intelligence grow quickly, intelligent transformation in education has become a major trend. This study suggests an intelligent educational resource allocation model based on multimodal feature fusion-driven (MERA) to fix the flaws with the current system, which are that it is static, not very responsive, and not very personalised. MERA combines the transformer structure, self-attention mechanism, and graph neural network (GNN) with multi-objective optimisation strategies to provide a detailed model and dynamic resource allocation for complicated, varied educational data. To fully test the models performance, three related experiments are planned and carried out. The results reveal that the MERA model is far better at using resources efficiently. In general, this study gives intelligent educational resource management a new technical path and a theoretical base. Keywords: multimodal feature fusion; intelligent educational resource allocation; transformer; graph neural network; GNN; multi-objective optimisation.
Abstract: Satellite remote sensing is crucial for large-scale, regular monitoring of mineral mining. It helps grasp the mining status and fight illegal activities. However, challenges like scarce training datasets, low detection efficiency, fragmented targets, and inaccurate positioning exist. This paper focuses on open-pit coal mines. It presents a saliency-guided image-cutting algorithm and an improved non-maximum suppression-based object-relocation algorithm. These are integrated with a deep-learning object-detection model to form a deep-learning-based mine-detection framework. Tests on 10 large-scale images show the framework achieves 85.22% recall and 45.73% precision efficiently, outperforming pure deep-learning models. Keywords: coal mine; open-pit mining area; deep learning; saliency detection; detection framework.
Abstract: The fusion of art using and artificial intelligence (AI) technology has revolutionised the creative landscape, introducing innovative techniques to produce and interpret visual art. AI has emerged as a powerful tool for generating hyper-realistic images and mimicking traditional art styles, raising profound questions about the authenticity and originality of artistic creations. As AI-generated photographs grow increasingly indistinguishable from human-made paintings. The research examines how advanced deep learning techniques enable accurate human vs. AI artwork differentiation through experimental model evaluations. Our research combined the previously trained VGG19 model with a specially developed CNN to discriminate between different image categories. The VGG19 model validated image feature extraction capabilities but the proposed CNN upgraded this performance with domain-based visual art recognition properties. Extensive testing of a curated AI-generated photograph and human-made painting dataset enabled the proposed CNN model to reach a 95% classification success rate, which outperformed the baseline VGG19 model results. Keywords: artificial intelligence; deep learning; art classification; computer vision; convolutional neural network; CNN; AI-generated images; artificial intelligence; photograph; painting. DOI: 10.1504/IJICT.2025.10072408
Abstract: Deep learning-based music generating techniques have slowly shown notable advancement in the area of popular music composition with the fast evolution of artificial intelligence technology. This work intends to look at how long-short-term memory (LSTM) networks are used in polyphonic pop music generation and their performance. An LSTM-based generative model is therefore created to properly catch the temporal dependencies in popular music and produce melodies and harmonies following the rules of music. Experimental findings indicate that, particularly in the coordination between several voices, the LSTM network can better preserve the harmony and consistency of the song when producing polyphonic music. At last, this study offers a perspective for future research considering the constraints of the present work; with the ongoing enhancement of dataset diversity and model optimisation, smart music composition will become more and more relevant in the domain of music composition. Keywords: LSTM networks; polyphonic music generation; popular music; temporal dependence. DOI: 10.1504/IJICT.2025.10072366
Abstract: Concerning the growing complexity of the business environment and career growth, conventional career planning strategies have become challenging to satisfy personal needs. This paper suggests a new career planning and path generating model based on multimodal learning technology, which integrates data from many sources (e.g., educational background, work experience, social networks, emotional data, etc.) so giving individuals more personalised and accurate advice. First, by preprocessing the data with feature fusion, the study creates tailored career path recommendations by building an adaptive model architecture combining deep learning and data mining approaches. In the experimental phase, the results reveal that the model has major benefits in terms of recommendation accuracy, personalisation and flexibility, and offers a fresh technical path for the field of career planning by means of the validation of the parameter tuning of the model and the effect of career path recommendation. Keywords: multimodal learning; career planning; path generation; personalised recommendation; data fusion. DOI: 10.1504/IJICT.2025.10072409
Abstract: In the context of the intelligent transformation of ideological and political education, how to achieve objective prediction of students' knowledge mastery has become a research difficulty. In response to the problem of insufficient model generalisation caused by traditional methods relying on non-public data, this study proposes a prediction model for ideological and political knowledge mastery based on deep tracing and knowledge graph (DKP model). Firstly, extract the temporal characteristics of learning behaviour and the logical correlation between knowledge points. Secondly, design a dynamic knowledge tracking framework and introduce the knowledge graph attention network (KGAT) to model the dialectical relationships and cognitive transfer paths between ideological and political knowledge points. Further, construct an interpretable mastery prediction index system. The cross-domain data fusion paradigm and open-source knowledge graph construction method proposed in the study provide a reproducible technical framework and infrastructure support for educational equity research. Keywords: ideological and political education; knowledge graph; LSTM; cognitive diagnosis. DOI: 10.1504/IJICT.2025.10072410
Abstract: The digital analysis and generation optimisation of traditional settlement landscape spatial forms is an important issue in the field of urban and rural cultural heritage protection. This study focuses on typical traditional settlements in Fujian where diverse cultures blend together, and proposes a GIS-based multidimensional data-driven method for generating and optimising landscape spatial forms. A spatial form iterative optimisation algorithm that integrates parameterised generation and generative adversarial networks with multi-objective genetic algorithms is developed to achieve intelligent generation and dynamic optimisation of traditional settlement spatial forms. Empirical research has shown that this method can effectively analyse the three-dimensional spatial morphology characteristics of typical types such as Minnan Red Brick Cuo settlements and Minxi Tulou settlements. The research results have important practical value for the dynamic inheritance of cultural heritage under the background of rural revitalisation. Keywords: geographic information system; generate adversarial networks; multi objective genetic algorithm; multidimensional data-driven approach; parameterised generation. DOI: 10.1504/IJICT.2025.10072365
Abstract: Text detection plays a vital role in applications like automated document analysis and scene understanding, yet achieving reliable accuracy in cluttered or low-contrast environments remains challenging. We propose FAF-Text, an English text detection framework that integrates adaptive feature filtering and multi-scale fusion to address these limitations. The filtering module employs gradient analysis to suppress noise and irrelevant patterns, while the fusion mechanism dynamically combines contextual and semantic features through attention-based learning. Evaluations on benchmark datasets demonstrate a 23% improvement in edge preservation and 18% enhancement in multi-scale recognition compared to existing methods. Ablation studies confirm the necessity of both modules, particularly under high-noise and low-resolution conditions, Furthermore, the framework's modular architecture ensures compatibility with multilingual OCR systems, offering a balance between computational efficiency and adaptability to complex text layouts. Keywords: English text; feature filtering; adaptive fusion; text detection. DOI: 10.1504/IJICT.2025.10072364
Abstract: In the era of media convergence, traditional news dissemination systems confront dual challenges of information entropy overload and diminished user engagement. This research proposes an intelligent propagation path optimisation framework leveraging interactive digital media, which integrates multimodal data perception with dynamic user behaviour feedback to establish a dual-layer reinforcement learning decision model. The methodology comprises three core components: a dynamic user interest quantification model combining temporal attention mechanisms with deep feature extraction, a Q-value iteration mechanism with entropy-constrained adaptive learning rate optimisation, and a multi-objective Pareto-optimal framework balancing coverage and timeliness under resource constraints. Keywords: interactive digital media; media convergence; dissemination path optimisation; reinforcement learning; information entropy. DOI: 10.1504/IJICT.2025.10072411 |