Forthcoming and Online First Articles
International Journal of Data Science
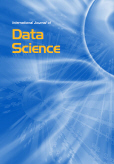
Forthcoming articles have been peer-reviewed and accepted for publication but are pending final changes, are not yet published and may not appear here in their final order of publication until they are assigned to issues. Therefore, the content conforms to our standards but the presentation (e.g. typesetting and proof-reading) is not necessarily up to the Inderscience standard. Additionally, titles, authors, abstracts and keywords may change before publication. Articles will not be published until the final proofs are validated by their authors.
Forthcoming articles must be purchased for the purposes of research, teaching and private study only. These articles can be cited using the expression "in press". For example: Smith, J. (in press). Article Title. Journal Title.
Articles marked with this shopping trolley icon are available for purchase - click on the icon to send an email request to purchase.
Online First articles are published online here, before they appear in a journal issue. Online First articles are fully citeable, complete with a DOI. They can be cited, read, and downloaded. Online First articles are published as Open Access (OA) articles to make the latest research available as early as possible.
Register for our alerting service, which notifies you by email when new issues are published online.
International Journal of Data Science (6 papers in press) Regular Issues
Abstract: With the rapid development of the economy, the problem of population aging has become increasingly prominent. In the current shortage of social endowment resources and imperfect endowment facilities, home-based endowment, as the most common endowment method, has played a great role in alleviating the pressure and contradiction of social endowment. However, the development time of home-based elderly care is relatively short, and the functionality and safety of its living environment are not suitable for the elderly, which is often difficult to meet the actual needs of the elderly. To effectively alleviate this dilemma, based on the analysis of the characteristics of the elderly, the demand for interior space design, and its development status, this paper has conducted effective research on the design and transformation of interior space for home-based care for the aged in combination with network security. To verify the effectiveness of network security, this paper has carried out the practice of interior space design and transformation and evaluated it from the three dimensions of functionality, security, and aesthetics. Keywords: Interior Space Design; Network Security; Home-based Pension; Elderly Group. DOI: 10.1504/IJDS.2025.10070395
Abstract: This article proposes an e-commerce platform-based Intelligent Financial Risk Blockchain Management under the Internet of Things (IFRBM-IoT) system to investigate and enhance the service quality, the standardisation of assurance processes inside a company, and the provision of means for achieving optimum security and quality control are all outcomes of a better profitability ratio risk management strategy. The framework uses the Neuro-fuzzy decision method with the control chart method to build an effective financial risk management system with Hyper ledger blockchain technology on an e-commerce platform, enabling in-depth analysis of financial risk evaluation and pledging greater data credibility, accountability, confidentiality, and integrity. The empirical data analysis findings demonstrate the model's high accuracy in data assessment and strong symmetric fit for managing financial risks inside the Internet business plan. The approach is practical for managing financial risks and assessing the effectiveness of data security measures. Keywords: Internet of Things; financial risk management; E-commerce; panel data regression analysis; Hyper ledger blockchain. DOI: 10.1504/IJDS.2025.10071212
Abstract: This study proposes a multilevel grey evaluation method integrated with machine learning to assess tourism resource (TR) development potential. Addressing challenges like poor management, resource scarcity, and unsustainable practices, the approach optimises resource allocation through an evaluation system analysing variation and grey weight vectors. Results show steady increases in evaluation weights over time, with average variation weight at 1.87 (total increase: 1.80) and grey weight at 0.50 (increase: 0.21). Compared to traditional systems, the optimised model improved service quality (9.92%), management level (10.25%), and government support (9.07%). This method enhances resource utilisation efficiency and promotes sustainable tourism development by identifying optimal strategies for TR exploitation. Keywords: Tourism Resource Development; Machine Learning; Multi-level Grey Method; Potential Evaluation. DOI: 10.1504/IJDS.2025.10071283
Abstract: Financial shared service centre is an emerging financial management method, which has been widely used in many multinational enterprises. This model can effectively improve the financial management efficiency of the enterprise, reduce the investment of human and material resources, and promote the optimal allocation of resources of the enterprise. Under the current market conditions, many problems have arisen in the traditional financial management model, such as low financial processing efficiency and high financial management costs. It is vital to assess its running perks in order to fully exploit its economic potential and to support and ensure the long-term growth of the business. The key to guaranteeing the long-term and stable growth of the monetary pooling centre is the deployment of transfer networks blockchain technology to construct a scientifically financial management system. The Financial Sharing Center's strategic goals are broken down into four categories: finances, customers, internal procedures, learning and development. The efficacy of the money sharing centre is assessed in this study using the fuzzy complete assessment method. Keywords: Financial Sharing; Deep Learning; Blockchain Technology; Performance Evaluation. DOI: 10.1504/IJDS.2025.10071763 A Commensurate Univariate Variable Ranking Method for Classification ![]() by Nuo Xu, Xuan Huang, Thanh Nguyen, Jake Yue Chen Abstract: To apply a variable ranking method for feature selection in classification, the notion of commensurateness is necessitated by the presence of different types of independent variables in a dataset. A commensurate ranking method is one that produces consistent and comparable ranking results among independent variables of different types, such as numeric vs categorical and discrete vs continuous. We invent a ranking method named Condition Empirical Expectation (CEE) and demonstrate it is the most commensurate among several representative ranking methods. Further, it has the highest statistical power as a test of independence when the categorical dependent variable is imbalanced. These properties make CEE uniquely suitable for fast feature selection for any datasets, especially those with high dimensionality of mixed types of variables. Its usage is demonstrated with a case study in facilitating preprocessing for classification. Keywords: variable types; variable ranking; variable relevance; commensurate; statistical dependence. DOI: 10.1504/IJDS.2025.10067405 Beauty Aids: can AI Improve Human behaviours with Imperfect Data? ![]() by Wenfeng Wang, Baizhou Xu, Bin Hu, Fuqing Li, Lalit M. Patnaik, Lujie Cui, Yunzhu Pan Abstract: This article aims to examine whether AI can improve human behaviors with imperfect data. Beauty aids with the pretrained AI model is taken as a practical example. This model integrated fuzzy reasoning with ResNet-50 for facial beauty prediction (FBP) and real-time recommendations of makeup behaviors. Results shown that the AI model can provide beauty aids for people whose facial data have not be included during the pretraining process and improve their makeup behaviors. The difference between the maximal and minimal values amounts to 33.62, implying that the effect of beauty aids is evident. The cross validation with perfect data further also confirmed that the effects of increased makeup experiences are worthy of further attention. The recommended degree of powder makeup for the volunteer is 0.118~0.2, while that of lipstick and blush makeup is 0.034~0.2. As an emerging technique, potential evolutions of the real-time beauty aids system with AI and data science will bring out the long-term future of FBP research. Keywords: models; parameters; data collection; makeup behaviors; facial beauty prediction. DOI: 10.1504/IJDS.2025.10070883 |