Forthcoming and Online First Articles
International Journal of Computational Intelligence in Bioinformatics and Systems Biology
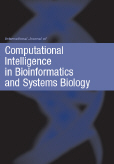
There are currently no forthcoming papers for this journal.
Register for our alerting service, which notifies you by email when new issues are published online.