Forthcoming and Online First Articles
International Journal of Computer Applications in Technology
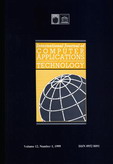
Forthcoming articles have been peer-reviewed and accepted for publication but are pending final changes, are not yet published and may not appear here in their final order of publication until they are assigned to issues. Therefore, the content conforms to our standards but the presentation (e.g. typesetting and proof-reading) is not necessarily up to the Inderscience standard. Additionally, titles, authors, abstracts and keywords may change before publication. Articles will not be published until the final proofs are validated by their authors.
Forthcoming articles must be purchased for the purposes of research, teaching and private study only. These articles can be cited using the expression "in press". For example: Smith, J. (in press). Article Title. Journal Title.
Articles marked with this shopping trolley icon are available for purchase - click on the icon to send an email request to purchase.
Online First articles are published online here, before they appear in a journal issue. Online First articles are fully citeable, complete with a DOI. They can be cited, read, and downloaded. Online First articles are published as Open Access (OA) articles to make the latest research available as early as possible.
Register for our alerting service, which notifies you by email when new issues are published online.