Forthcoming and Online First Articles
International Journal of Computational Materials Science and Surface Engineering
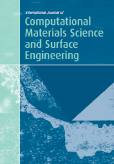
There are currently no forthcoming papers for this journal.
Register for our alerting service, which notifies you by email when new issues are published online.